Another data issue in our series of Industrial IoT data problems is that of Latent Data. High latency is limiting productivity for industrial companies across the globe.
Simply put, latent data is data that doesn’t show up quickly enough to have value. Or, at the very least, its value is diminished. Even if sensors and devices are functioning correctly, field data may not make it upstream – where operations or business decisions are made – in time for processing. The data may reflect reality and tell a cohesive story, but high latency caused by network issues or processing limitations prevent it from reaching those who need it most.
Latent data can be frustrating for industrial companies, as they’ve invested heavily in their digital infrastructure and capabilities, only to miss out on the real value of digitization. By deploying hundreds or thousands of interconnected devices to track operational processes, companies should be able to monitor infrastructure with tremendous efficiency. Latent data takes away from this purpose and hinders decision-making.
Latent data is not an issue of data quality, like bad data (link to post), but rather one of data accessibility and usefulness. Latent data is “good” intelligence; it could be put to use if the data wasn’t delayed. Solving latent data problems, or “unclogging” data streams, empowers field workers, analysts, and leaders to manage their assets more effectively over the long term.
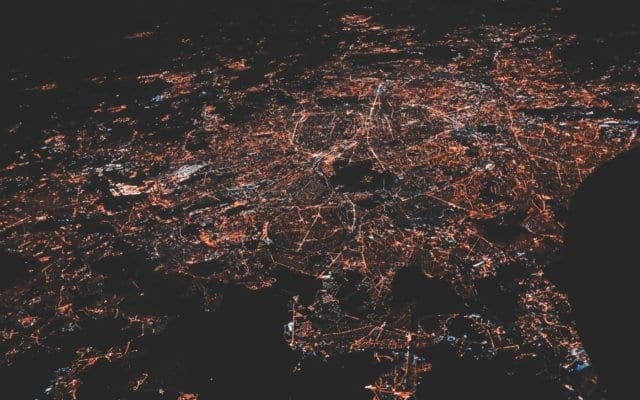
As the world creates more and more data, network latency is a growing concern. Photo by Anastasia Dulgier
What causes latent data?
Latent data problems are frequently caused by outdated network design techniques and limited resources.
Traditionally, legacy SCADA systems have relied on flat network designs to minimize the number of routers and switches needed across networks. Under this configuration, operational data collected on the fringes traveled along predefined routes to databases or visualization clients.
As devices were added to flat networks, data pipelines became increasingly clogged, causing delays in transmission. To compensate for these latency issues, network technicians would often minimize polling frequency. However, this strategy would lead to another data problem – low-resolution data.
More modern SCADA systems employ a hybrid approach and push IP-based, packet-switching protocols to the edge. By doing so, network technicians create additional paths for data to travel once it reaches critical transmission points.
But, data can still get jammed at the edge. Something as simple as a gateway losing power or connectivity can cause latency problems. Additionally, too many hops in a packet-switched network can also lead to delayed data.
Although some physical transport media can alleviate latency issues, most industrial companies with remote assets don’t have the financial resources to execute significant investment in private, IP-based networks with high-speed physical transport media. Installing fiber or licensing dedicated bandwidth are both costly endeavors. As a result, many businesses have to choose between deploying low-speed networks, or leasing capacity from public communications infrastructure.
The downside with this approach is that large-scale environmental issues, such as natural disasters or public health emergencies, can quickly overwhelm public networks. Data bursts can easily exceed bandwidth capacity if networks aren’t optimized, leading to information and value leakage. And it doesn’t have to just be a freak occurrence; many industrial companies don’t know how to choose networks plans to secure private APNs or prioritized traffic in a world where more and more people are relying on public communication.
Latency issues can also compound as data moves from the edge to network applications. For example, if data arrives late to a processing point, such as a gateway or analytics engine, it can delay subsequent transmissions. In other words, upstream latency issues can have downstream ripple effects, much in the same way that accidents or over-braking earlier in a day can cause massive traffic jams hours later.
Who is affected by latent data?
Field operations workers are most impacted by the latent data problem.
These individuals are responsible for making rapid, tactical decisions based on intel from remote assets. Every second between an alarm-worthy event and the alarm being seen can have severe effects on environmental, safety, and financial performance.
Consider a scenario involving a major oil spill. A massive interstate pipeline transporting 500,000 barrels of oil per day could leak 5,000 barrels (worth ~$250,000) in just 15 minutes. In this situation, latency would be tremendously damaging on multiple fronts. The longer it takes for field workers to receive an alert and respond, the more oil that spills onto the ground.
Field workers must be able to see anomalous data as soon as it’s generated so that they can act quickly and accordingly. And fixes may not be as straightforward as replacing faulty sensors. Latency issues may exist at a higher level and require broader reconfiguration or bandwidth capacity improvements.
Further downstream, office-based analysts can struggle to glean important insights if they don’t receive data in time. The traffic jam effect mentioned previously applies here. Data becomes more delayed the further up the chain it travels. Those who perform large-scale data aggregations can’t process information quickly if it’s delayed, which affects executive decision-making power.
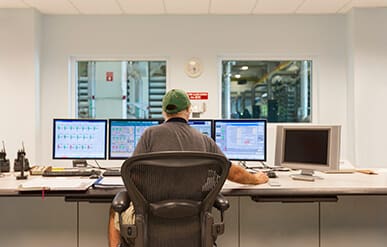
Operations personnel can’t make decisions on time with latent data.
What do we need to consider?
As alluded to previously, system designers should be aware of the minimum network capacity required to support existing infrastructure at its busiest times. They should also consider future expansion efforts and what it would take to enable new devices on their networks without running into bandwidth issues down the road. Those who employ legacy, unlicensed transport media typically suited for high latency data transport must update to lighter-weight protocols, like MQTT, that don’t cause as many bottlenecks for data flow.
Latency issues may also be hidden by compensating elements within the network. For example, a company might want (or need) to get data from a sensor every 15 seconds, but because of bandwidth issues that would cause latency, they only get data every 5 minutes. So, the underlying latency issue might be solved, but the network technicians now have a low-resolution data problem – a problem we will discuss in a future post!
The rise of edge computing power may also alleviate high latency issues for some industrial companies with distributed infrastructure. Since networking costs are not decreasing faster than data creation is increasing, IoT engineers have to continue to find creative ways to move processing to the edge to reduce demand on their networks.
Finally, industrial companies should consider what opportunities might be available to place IoT devices on prioritized networks to minimize bandwidth shrinkage during high-traffic times. These networks offer capacity advantages, as well as protection during outages.
Networks like the government-mandated FirstNet network, which was initially designed for First Responders, could be options for some industrial companies. FirstNet has extended support beyond emergency personnel to include critical infrastructure and critical manufacturing IoT devices. Industrial companies should consider if they could qualify for this type of networking infrastructure.
What’s at stake for your business?
Here are a few considerations related to the latent data problem:
- What are your “just-in-time” requirements related to data?
- Where are you compensating for latent data by accepting low-resolution data?
- Do you have a baseline understanding of when data should arrive?
- Is your network configured to meet current – and future – bandwidth needs?
- What is the maximum amount of data your network can transport? And how does this affect your ability to change business processes?
- Can you recognize latent data and tie it to the responsible devices?
Use these questions to help evaluate your preparedness related to latency issues across your deployments.
With WellAware, solving any IoT data problem is easy, as our team understands what challenges industrial organizations face when piloting and scaling digital projects. To learn more about how we work, contact us today.
Check back in soon to read our next Data Problem blog series post: “Siloed” Data!
Recent Comments